AI and Tools Give Data—But Do You Really Understand It?
In today’s tech-driven business environment, artificial intelligence (AI) and automated tools have become essential for decision-making in areas like cloud management, cybersecurity, and data analysis. These tools generate a wealth of information and insights, giving businesses access to data at an unprecedented scale.
But here’s the question: Are you really equipped to interpret that data?
While AI and tools provide valuable insights, they can also create a false sense of security. Data is only as useful as the expertise applied to interpret it. Misunderstanding or misinterpreting that data can lead to misguided decisions, wasted resources, and overlooked critical issues. This article explains why relying on AI and tools alone is risky and why expert analysis is essential.
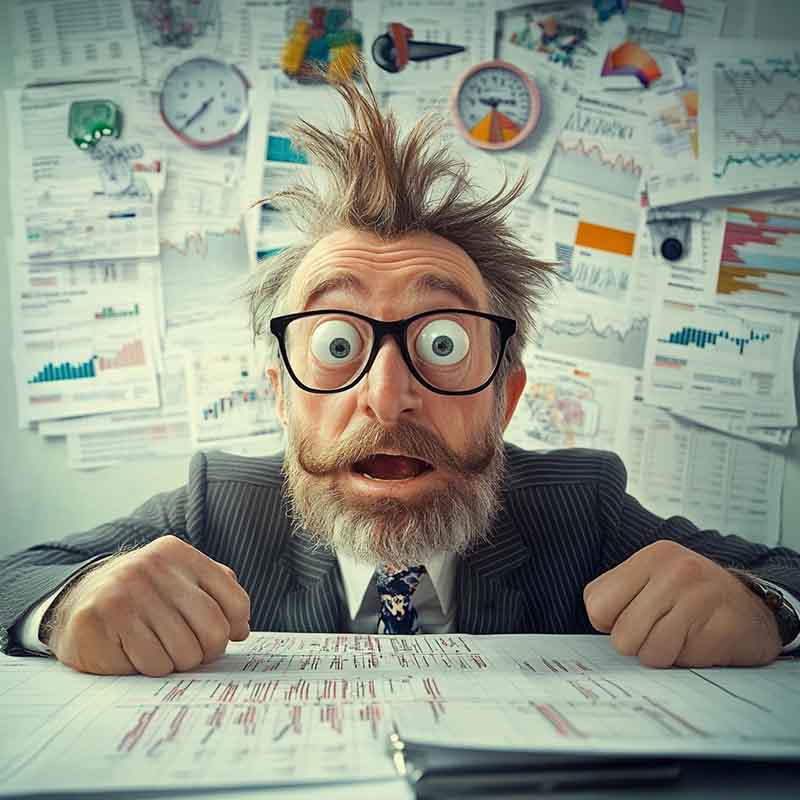
The Risks of Misinterpreting Data from AI and Tools
AI and tools are designed to flag potential issues based on predefined rules and algorithms. However, the data they generate often lacks context. This is especially true in fields like cloud computing or cybersecurity, where a flagged issue may not be the root cause of a problem, but simply a symptom.
Without the right expertise, businesses can quickly fall into the trap of interpreting these signals incorrectly, leading to changes that don’t address the underlying problem. This can cause inefficiencies, bottlenecks, and resource wastage over time.
Overlooking Real Problems
When businesses focus on fixing what the tools flag as issues, they can easily overlook deeper, more systemic problems. AI and automated tools can only identify symptoms—they are not designed to diagnose the root cause of complex tech challenges.
For example, a tool might alert a company about increasing CPU usage in its cloud infrastructure. But without a thorough understanding of why the CPU usage is high, the business might simply increase cloud resources, thinking the problem is solved. The real issue, however, could be an inefficiency in how applications are handling data or unnecessary processes consuming resources. Without that deeper analysis, the business is only treating the symptom, not the cause.
Spiralling into Poor Decisions
Misinterpreting data often leads to a series of poor decisions that compound over time, resulting in a spiral of ineffective changes. Here’s how this process can unfold:
- A Tool Flags an Issue: A cloud management tool detects that your CPU usage is high or that storage is nearing its limit.
- You Act Based on Partial Information: Believing that the tool’s flagged issue is the root cause, you increase resources—investing in more cloud capacity or additional storage—without investigating the true source of the problem.
- The Problem Persists: Despite scaling resources, the problem continues. The real issue, such as a misconfigured application or redundant data, goes undetected because your focus was on the tool’s flag rather than the broader context.
This leads to a cycle where businesses throw money and resources at what they perceive to be the problem, without ever addressing the true cause. As a result, inefficiencies grow, operational costs rise, and the long-term strategy gets derailed.
Why does this happen?
Because tools like AI and automated monitoring systems present data points in isolation. They don’t consider the broader architecture of your system or how different parts of your infrastructure interact with each other. AI is great at pattern recognition—it can detect anomalies, but it cannot explain why those anomalies exist or what they mean in the larger context.
Do You Have the Expertise to Interpret AI Data?
This is the central question. Many businesses trust their tools and feel that they are equipped to act based on the data provided. But in many cases, this confidence is misplaced. Simply having data in front of you does not mean you have the context or the understanding to make the right decision.
For example, cybersecurity tools might detect a vulnerability in your network. However, vulnerabilities come in different forms—some are minor and pose little threat, while others can be catastrophic. Do you have the expertise to know the difference? Can you assess whether this vulnerability is a false positive, a manageable risk, or a critical issue that requires immediate attention?
The same applies to cloud performance monitoring. A spike in traffic or a rise in resource usage could be a sign of normal operations or an indicator of an underlying problem. Without the expertise to dig deeper, you could make decisions that lead to higher costs or, worse, a reduction in your system’s performance and efficiency.
The Real Risks of Overconfidence
When you assume you are equipped to interpret data, the risks are significant. Misinterpreting data from AI and tools can lead to:
- Wasted resources: Investing in more cloud capacity or cybersecurity measures based on false positives.
- Missed opportunities: Failing to see the real problem because you’re focused on symptoms rather than the root cause.
- Reduced performance: Implementing changes that actually degrade system performance rather than improving it.
- Long-term impact: Poor decision-making today can compromise your entire tech strategy over the long term, affecting your business’s scalability, security, and innovation.
A great example is cloud management. A tool might recommend expanding storage due to increased usage, but without expert input, businesses may not realise that old or unnecessary files are contributing to the increased demand. The business ends up spending money on more storage when a simple clean-up of old data would have solved the problem at a fraction of the cost.
AI and Tools Are Powerful, But Expertise is Essential
AI and tools provide invaluable data and insights, but they are not substitutes for human expertise. The data they present can be compelling, but it is only part of the story. Without expert analysis to put the data in context, businesses risk making decisions that waste resources, ignore critical problems, and ultimately hurt long-term strategy.
Before acting on the data provided by AI and tools, businesses need to ask themselves: Do we have the expertise to interpret this data? If not, it’s time to bring in experts who can turn that data into actionable insights. Only with the right balance of technology and expertise can businesses ensure that their decisions lead to growth, efficiency, and sustained success.